Enhancing Your Shopify Store with Machine Learning Product Recommendations.
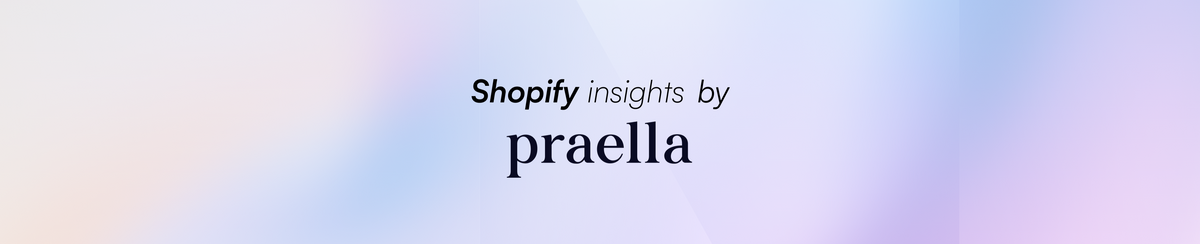
Table of Contents
- Introduction
- Understanding Shopify ML Product Recommendations
- Types of Recommendation Engines
- Benefits of ML Product Recommendations in Shopify
- Implementing Shopify ML Product Recommendations
- Case Studies: Learning from Praella’s Successes
- Conclusion
- FAQ
Introduction
Imagine walking into a physical store where every shelf is tailored precisely to your tastes. Wouldn’t that make shopping irresistible? For online retailers, replicating this personalized experience digitally is the goal, and Shopify's machine learning (ML) product recommendations provide the key. This post dives into how integrating machine learning product recommendation engines into your Shopify store can transform the customer experience and boost sales.
Shopify, a leader in e-commerce platforms, enables businesses to adopt these cutting-edge recommendation systems to draw customers to products they're genuinely interested in. As we unravel the intricacies of Shopify ML product recommendations, we’ll explore their significance, strategies for implementation, and potential impacts on your bottom line.
This blog will cover the types of recommendation engines, provide strategies to enhance them, and offer insights into Praella’s services, which align with optimizing these technologies for your e-commerce success.
Understanding Shopify ML Product Recommendations
E-commerce product recommendations are essentially personalized nudges that guide online shoppers toward potentially desirable products. Through tailored suggestions based on a customer’s browsing and purchasing history, these recommendations have an impressive ability to increase sales and enhance user experience.
The core of these personalized experiences lies in recommendation engines powered by machine learning algorithms. These engines analyze vast amounts of user data to deliver suggestions that feel intuitive and natural to the shopper. As a Shopify store owner, integrating such capabilities can lead to a significant boost in average order value and customer loyalty due to its personalized nature.
Types of Recommendation Engines
Harnessing the full potential of ML product recommendations involves understanding the different types of engines available:
1. Collaborative Filtering
Collaborative filtering uses data from multiple users to make recommendations based on similarities. It comes in two forms:
- User-Based Filtering: Recommends products based on what similar users have liked or purchased.
- Item-Based Filtering: Suggests items based on their similarity to products the user has previously engaged with.
2. Content-Based Filtering
This approach leverages the attributes of a product to suggest similar items. By analyzing product descriptions and features the user has shown interest in, the system builds a model of preferences to highlight compatible options.
3. Hybrid Systems
Combining collaborative and content-based filtering, hybrid models overcome individual limitations to provide a more holistic recommendation approach. This flexibility allows them to adapt and refine suggestions in a more responsive manner.
Benefits of ML Product Recommendations in Shopify
Adopting machine learning recommendation engines offers several compelling advantages for e-commerce owners:
Increase in Sales and Revenue
Personalized recommendations directly influence purchase decisions, potentially increasing revenues. According to various studies, a large portion of sales on platforms like Amazon stems from these engines strategically suggesting items.
Improved User Experience
Shopping becomes more engaging and fulfilling when users quickly find relevant products. Personalized pathways lead to smoother navigation, enhancing overall satisfaction and encouraging repeat visits.
Enhanced Customer Loyalty
Repeated positive interactions through tailored recommendations contribute to long-term customer loyalty. Personalized experiences create a bond with the brand, motivating customers to return.
Optimized Marketing Spend
By understanding customer preferences, businesses can better allocate resources. Enhanced ad targeting and inventory management inform better marketing strategies, optimizing the effectiveness of promotional efforts.
Data Insights for Continuous Improvement
The insights garnered from recommendation systems offer a wealth of information about consumer behavior and preferences. This data aids strategic decision-making and future product development.
Implementing Shopify ML Product Recommendations
To harness these benefits, thoughtful implementation is essential. Here are some strategic approaches:
Leverage Customer Data
Use both historical and real-time data to understand customer behavior. Tools like Amazon Personalize can enhance this capability, supporting a fuller view of user interactions and preferences.
Personalize Across Touchpoints
Ensure personalized recommendations appear at every customer touchpoint - from homepage to checkout. This ensures customers consistently encounter relevant suggestions.
Integrate with Physical and Digital Channels
For businesses with physical and online stores, using data from both can inform better product recommendations. For instance, linking in-store purchases to online suggestions creates a unified shopping experience.
Optimize Through Continuous Testing
Regularly test and tweak your recommendation algorithms. A/B testing different strategies can help identify which approaches best enhance conversion rates and customer satisfaction.
Case Studies: Learning from Praella’s Successes
At Praella, we've assisted multiple clients in transforming their online platforms using cutting-edge solutions, such as machine learning product recommendations:
- DoggieLawn: Migrating from Magento to Shopify Plus with a focus on recommendations resulting in a 33% increase in conversions (Read more).
- Pipsticks: For this vibrant brand, Praella crafted an exciting online platform that echoed its energetic spirit with tailored recommendations (Read more).
Conclusion
Machine learning product recommendations on Shopify are indispensable for modern e-commerce. By delivering personalized shopping experiences that cater directly to consumer interests, businesses can drive substantial growth in sales and customer retention.
For Shopify merchants eager to integrate these advanced recommendation systems, collaborating with experts like Praella can streamline the process. From user experience and design to strategic growth and continuity, Praella supports transformative e-commerce solutions that can elevate your brand to new heights. Explore these possibilities by engaging with Praella’s offerings, ensuring your store leads with innovation and excellence in personalized recommendations.
FAQ
What are the primary types of product recommendation engines used in e-commerce platforms?
The main types include collaborative filtering (user-based and item-based), content-based filtering, and hybrid systems combining both approaches.
How do machine learning recommendations affect customer loyalty?
By providing personalized recommendations that enhance shopping convenience, customers feel understood and catered to, thereby fostering loyalty and encouraging repeat purchases.
Can Praella help with integrating machine learning recommendation systems on my Shopify store?
Yes, Praella specializes in developing scalable and innovative e-commerce solutions. We can assist in integrating these systems effectively to enhance your store's performance. You can learn more about their services here.
Why should I choose a hybrid recommendation system?
Hybrid systems offer a comprehensive approach by combining the strengths of both collaborative and content-based filtering, thus providing more accurate and adaptable product recommendations.